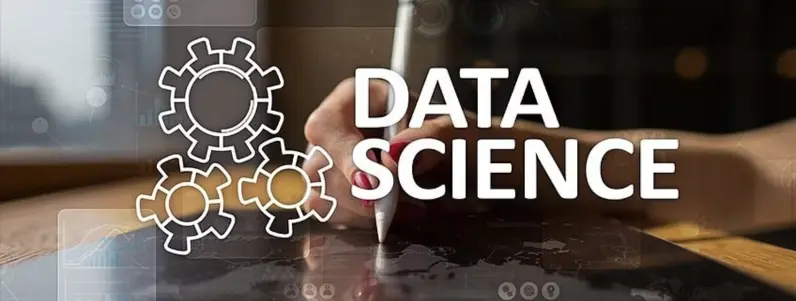
Introduction to Data Science
Data wisdom is a multidisciplinary field that combines colorful ways, tools, and methodologies to prize precious perceptivity and knowledge from data. It involves the operation of mathematics, statistics, programming, advanced analytics, artificial intelligence( AI), and machine literacy to uncover retired patterns, trends, and connections within large and complex datasets.
The accelerating growth in data sources has made data wisdom one of the swift-growing fields across diligence. Organizations are decreasingly counting on data scientists to dissect and interpret data, furnishing practicable recommendations that drive informed decisions- timber and strategic planning.
The data wisdom lifecycle encompasses several stages, starting from data collection and ingestion, followed by data storehouse and processing, exploratory data analysis, modeling, and eventually, communication of perceptivity through reports and visualizations. Each stage involves specific ways and tools acclimatized to handle the challenges and complications associated with data.
Data scientists play a pivotal part in this process, exercising their moxie to navigate through vast quantities of data, excerpt meaningful information, and develop prophetic models that can be used to break complex problems and drive invention. They retain a combination of logical chops, sphere knowledge, and programming proficiency to effectively dissect and interpret data, turning it into practicable perceptivity that significantly impacts business issues.
In summary, data wisdom is a vital discipline that harnesses the eventuality of data to uncover precious perceptivity, and drive decision- timber, and companion associations toward achieving their pretensions. It has become an integral part of colorful diligence, revolutionizing the way businesses operate and introducing it in the ultramodern data-driven period.
Data science vs. machine learning
Data wisdom and machine literacy are nearly affiliated fields, but they've distinct focuses and objects. Then is a breakdown of their differences
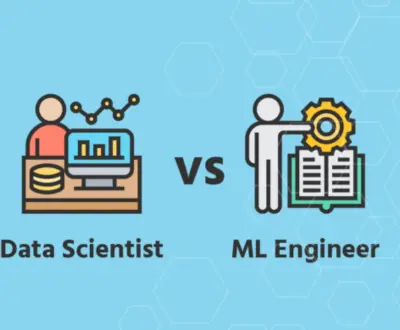
- Data Science
- Machine Learning
Data wisdom is a multidisciplinary field that involves rooting perceptivity, patterns, and knowledge from data. It encompasses colourful ways similar as data cleaning, data preprocessing, data analysis, statistical modeling, and visualization. The thing of data wisdom is to discover meaningful information and make informed opinions grounded on data. Data scientists use programming languages like Python or R, along with tools and libraries, to reuse and dissect data. They may work on problems similar to data mining, prophetic modeling, recommendation systems, and data-driven decision- timber.
Machine literacy is a subfield of artificial intelligence that focuses on the development of algorithms and models that enable computers to learn patterns from data and make prognostications or opinions without unequivocal programming. Machine literacy algorithms can be distributed into three main types supervised literacy, unsupervised literacy, and underpinning literacy. Supervised literacy uses labeled training data to make prognostications, unsupervised literacy finds patterns in unlabeled data, and underpinning literacy involves literacy from commerce with a terrain to maximize prices. Machine literacy ways are used to make models that can perform tasks similar to image recognition, natural language processing, fraud discovery, and recommendation systems.
In summary, data wisdom is a broader field that involves working with data to prize perceptivity and break problems using colorful ways, including machine literacy. Machine literacy, on the other hand, is a specific approach within data wisdom that focuses on developing algorithms and models to enable computers to learn from data and make prognostications or opinions. Machine literacy is one of the tools employed by data scientists to dissect and interpret data, but it isn't the sole focus of data wisdom.
Data science and analytics
Data wisdom and analytics are two nearly affiliated fields that partake in common pretensions but differ in their approaches and compass. Then is a comparison between data wisdom and analytics
- Data Science
- Analytics
Data wisdom is a multidisciplinary field that combines statistical analysis, machine literacy, data visualization, and sphere moxie to prize perceptivity and knowledge from data. Data scientists use a combination of programming chops, statistical knowledge, and business wit to uncover patterns, make prognostications, and decide meaningful perceptivity from large and complex datasets. They employ colorful ways similar to data drawing, preprocessing, exploratory data analysis, statistical modeling, and machine literacy to dissect and interpret data. Data wisdom frequently involves the use of programming languages like Python or R, as well as tools and libraries for data manipulation, analysis, and visualization. The ultimate thing of data wisdom is to decide practicable perceptivity and make data-driven opinions.
Analytics, in a broader sense, refers to the process of discovering, interpreting, and communicating meaningful patterns and perceptivity from data. It involves collecting, organizing, and assaying data to gain perceptivity and inform decision- timber. Analytics can be performed using colorful ways, including statistical analysis, descriptive analytics, individual analytics, prophetic analytics, and conventional analytics. The focus of analytics is frequently on understanding once and present data trends, relating patterns, and answering specific business questions. It can involve working with structured and unshaped data, and the ways users can range from introductory data summarization to sophisticated statistical modeling. Analytics can be applied to colorful disciplines, similar as marketing analytics, fiscal analytics, healthcare analytics, and more.
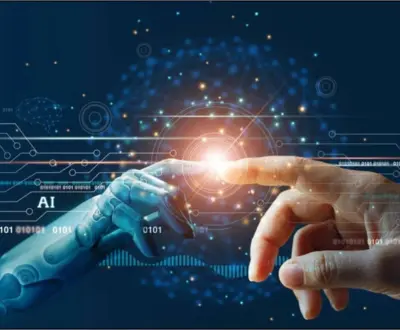
In summary, data wisdom is a more comprehensive and advanced field that encompasses a broader range of ways, including analytics. Data wisdom involves rooting perceptivity from data using statistical analysis, machine literacy, and other advanced styles, while analytics focuses on using data to gain perceptivity and inform decision- timber. Analytics can be considered a subset of data wisdom that emphasizes the use of statistical ways and data analysis to answer specific questions and break business problems.
Case Studies
- Case 1 : Automation of Parking System and Improving Parking efficiency
- Case 2 : AI for Education
- Case 3: BI for Loans of NBFC